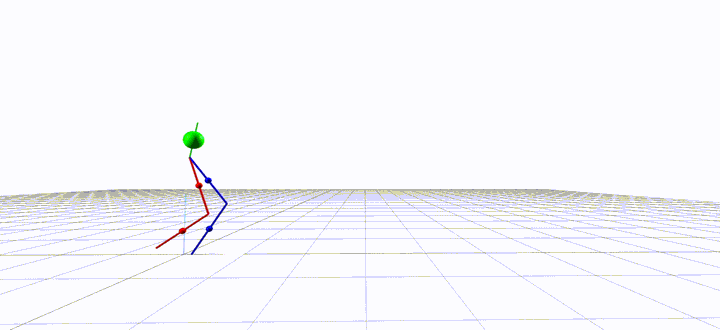
Our project is aimed at trajectory optimization in bipedal robotics, specifically in three fundamental gaits: hopping, walking, and running. The central challenge we address is the computational complexity often encountered in multi-step trajectory optimizations. Typically, these optimizations yield gaits that do not align with natural human movements, a gap that our work aims to bridge.
We’ve developed the Gait Modularization Optimization Technique (GMOT) to generate more natural gait trajectories efficiently. GMOT stands out for its unique two-loop approach. In the inner loop, we focus on designing and optimizing a repeatable gait unit, applying symmetry constraints to the initial and final states for consistency and efficiency. In the outer loop, these optimized gait units serve as a foundation for optimizing the full gait trajectory.
Our method, GMOT, has demonstrated remarkable results. It not only aligns more closely with human-like movement patterns but also significantly reduces the time required for solving complex trajectory optimization problems compared to traditional methods with naive initialization. In essence, GMOT is set to transform the way we approach bipedal robotic movement, making it more efficient, stable, and, importantly, more akin to natural human motion.