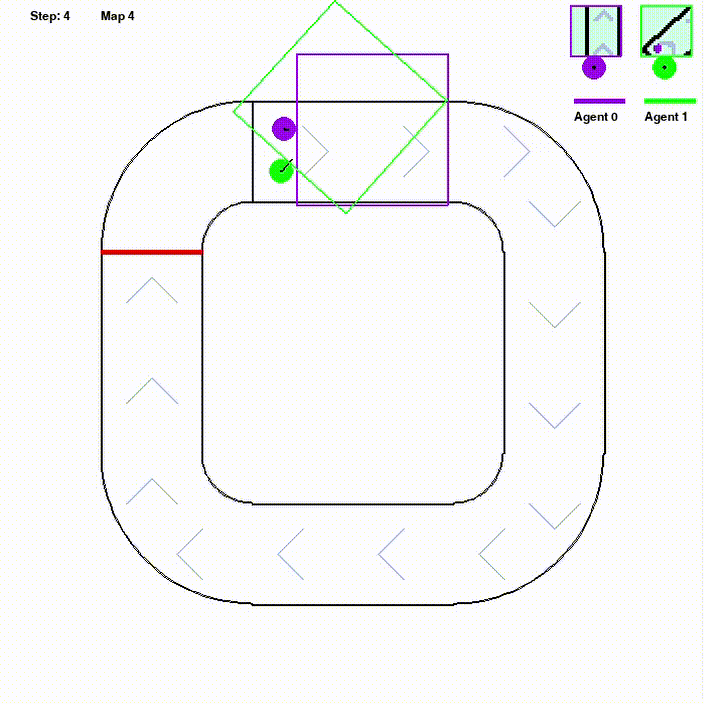
Regular Multi-agent Reinforcement Learning methods are not likely to perform well on environments with sparse rewards or integrated task settings. We expected to explore a heuristic reward function and high-capacity data replay framework to tackle this problem. We mainly study on Olympics Running environment, in which the main challenge is how to perform well in different environments with little positive feed- back with a single policy. Using models based on PPO, we construct a parallel framework with efficient data sampling. To deal with the sparse reward, reward-shaping approaches inspired by robotics path planning algorithms called AStar trace potential is utilized. In refining the learning of the reward, we develop an end-to-end routine with the mechanism of curiosity, which performs well on the framework below. Self-play training is also utilized in training routine to achieve better leaning on multi-agent task.
My role is to enhance PPO algorithm with a curiosity-driven approach, yielding a performance boost of 50% over the $A^*$-inspired reward shaping in multifaceted scenarios. My curiosity-driven agent achieves the best results in our project, wth a 62% win rate against Jidi PPO baseline and second place in class rankings.